Molecular Descriptors For Cheminformatics Pdf To Excel
Molecular descriptors play a fundamental role in chemistry, pharmaceutical sciences, environmental protection policy, and health researches, as well as in quality control, being the way molecules, thought of as real bodies, are transformed into numbers, allowing some mathematical treatment of the chemical information contained in the molecule. This was defined by Todeschini and Consonni as:
- Molecular Descriptors For Cheminformatics Pdf To Excel Pdf
- Molecular Descriptors For Cheminformatics Pdf To Excel Online
- Molecular Descriptors For Chemoinformatics Pdf To Excel
- Molecular Descriptors For Chemoinformatics Pdf To Excel Free
Molecular descriptors for chemoinformatics (2 volumes). Toward the ubiquitous use of Cheminformatics and QSAR modeling. Thousands of molecular descriptors. UCSF chemViz2 is a Cytoscape app that extends the capabilities of Cytoscape into the domain of cheminformatics. ChemEngine: harvesting 3D chemical structures of. An open workflow environment for cheminformatics. ‘plug and play’ cheminformatics toolkit With over 1000 API functions, Accord SDK lets. 100 molecular descriptors & properties can be calculated from 2D structure, giving you the power. Excel - adding all the functionality available in Accord into MS. Cheminformatics Statistical mechanics Molecular mechanics Semi-empirical methods Ab initio quantum chemistry All these methods, except the last, rely on empirical information (parameters, energy levels etc.). In this course we will focus on the last of these methods. Such as contained in molecular descriptors. A Java framework for cheminformatics. As a result, it is possible to read in a variety of molecular formats, calculate molecular descriptors and evaluate fingerprints. In addition, we describe. 4 Chemical Informatics Functionality in R Function Description.
'The molecular descriptor is the final result of a logic and mathematical procedure which transforms chemical information encoded within a symbolic representation of a molecule into a useful number or the result of some standardized experiment.'[1]
By this definition, the molecular descriptors are divided into two main categories: experimental measurements, such as log P, molar refractivity, dipole moment, polarizability, and, in general, additive physico-chemical properties, and theoretical molecular descriptors, which are derived from a symbolic representation of the molecule and can be further classified according to the different types of molecular representation.
The main classes of theoretical molecular descriptors are: 1) 0D-descriptors (i.e. constitutional descriptors, count descriptors), 2) 1D-descriptors (i.e. list of structural fragments, fingerprints),3) 2D-descriptors (i.e. graph invariants),4) 3D-descriptors (such as, for example, 3D-MoRSE descriptors, WHIM descriptors, GETAWAY descriptors, quantum-chemical descriptors, size, steric, surface and volume descriptors),5) 4D-descriptors (such as those derived from GRID or CoMFA methods, Volsurf).
Invariance properties of molecular descriptors[edit]
The invariance properties of molecular descriptors can be defined as the ability of the algorithm for their calculation to give a descriptor value that is independent of the particular characteristics of the molecular representation, such as atom numbering or labeling, spatial reference frame, molecular conformations, etc. Invariance to molecular numbering or labeling is assumed as a minimal basic requirement for any descriptor.
Two other important invariance properties, translational invariance and rotational invariance, are the invariance of a descriptor value to any translation or rotation of the molecules in the chosen reference frame. These last invariance properties are required for the 3D-descriptors.
Degeneracy of molecular descriptors[edit]
This property refers to the ability of a descriptor to avoid equal values for different molecules. In this sense, descriptors can show no degeneracy at all, low, intermediate, or high degeneracy. For example, the number of molecule atoms and the molecular weights are high degeneracy descriptors, while, usually, 3D-descriptors show low or no degeneracy at all.
Basic requirements for optimal descriptors[edit]
- Should have structural interpretation
- Should have good correlation with at least one property
- Should preferably discriminate among isomers
- Should be possible to apply to local structure
- Should possible to generalize to 'higher' descriptors
- Should be simple
- Should not be based on experimental properties
- Should not be trivially related to other descriptors
- Should be possible to construct efficiently
- Should use familiar structural concepts
- Should change gradually with gradual change in structures
- Should have the correct size dependence, if related to the molecule size
See also[edit]
References[edit]
- ^Roberto Todeschini and Viviana Consonni, Handbook of Molecular Descriptors, Wiley-VCH, 2000.http://www.moleculardescriptors.eu/books/handbook.htm
Bibliography[edit]
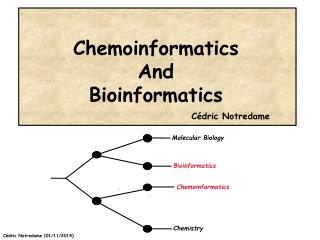
Roberto Todeschini and Viviana Consonni, Molecular Descriptors for Chemoinformatics (2 volumes), Wiley-VCH, 2009.
Mati Karelson, Molecular Descriptors in QSAR/QSPR, John Wiley & Sons, 2000.
Fsx a321 spirit. James Devillers and Alexandru T. Balaban (Eds.), Topological indices and related descriptors in QSAR and QSPR. Taylor & Francis, 2000.
Lemont Kier and Lowell Hall, Molecular structure description. Academic Press, 1999.
Alexandru T. Balaban (Ed.), From chemical topology to three-dimensional geometry. Plenum Press, 1997.
External links[edit]
- March 22, 2019
- Wu, Tong-Yng
- Affiliation: School of Medicine, UNC/NCSU Joint Department of Biomedical Engineering
- The increasing availability of biological and chemical data has led to a critical need for cheminformatics and bioinformatics tools to analyze the data. However, not all datasets contain sufficient information for significant analysis. One problem is High Dimension Low Sample Size (HDLSS), where the number of structural characteristics, e.g., molecular descriptors, that can be calculated from a single compound (high dimensionality) far exceeds the number of compounds (low sample size). A major challenge associated with modeling HDLSS data is overfitting, and specialized tools are required to overcome the statistical difficulties inherent to HDLSS. We improved the Distance Weighted Discrimination (DWD) statistical learning method through a new variable selection technique for estimating the intrinsic dimension of a dataset, i.e., the minimum number of descriptors to classify data. Compared to SVM and DWD without variable selection, DWD with variable selection achieved higher prediction accuracy on several benchmarking datasets and allowed the identification of key molecular features that contribute to investigated biological properties, e.g., inhibition of AmpC β-lactamase and binding affinity for the various serotonin receptors. For analyzing and modeling stereochemistry-dependent datasets, we developed chiral atom-pair descriptors (3D chiral atom-pair), which were calculated from three-dimensional molecular structures. QSAR models built with these descriptors, versus either 3D non-chiral atom-pair or 2D Dragon descriptors, more accurately predicted antimalarial activity and binding affinities of small molecules toward various receptors. Our method not only led to the identification of a subset of chiral atoms that are expected to affect the selected biological property, e.g., antimalarial activity, but also enabled the development of models that would not be possible otherwise. To aid automatic protein function annotation, especially in the case of functional homologs, we developed new protein descriptors based solely on protein's structure. Our method showed sensitivity comparable to that of ScanPROSITE. When predicted annotations from both ScanPROSITE and our method were combined into a consensus model, we observed a significant gain in prediction reliability and the successful functional annotation of proteins with low sequence similarity.
- August 2012
Molecular Descriptors For Cheminformatics Pdf To Excel Pdf
Molecular Descriptors For Cheminformatics Pdf To Excel Online
- In Copyright
- Lalush, David Scott
- Doctor of Philosophy
Molecular Descriptors For Chemoinformatics Pdf To Excel
- 2012